Sports
How Our March Madness Predictions Work – 538 — Election Polls, Politics …
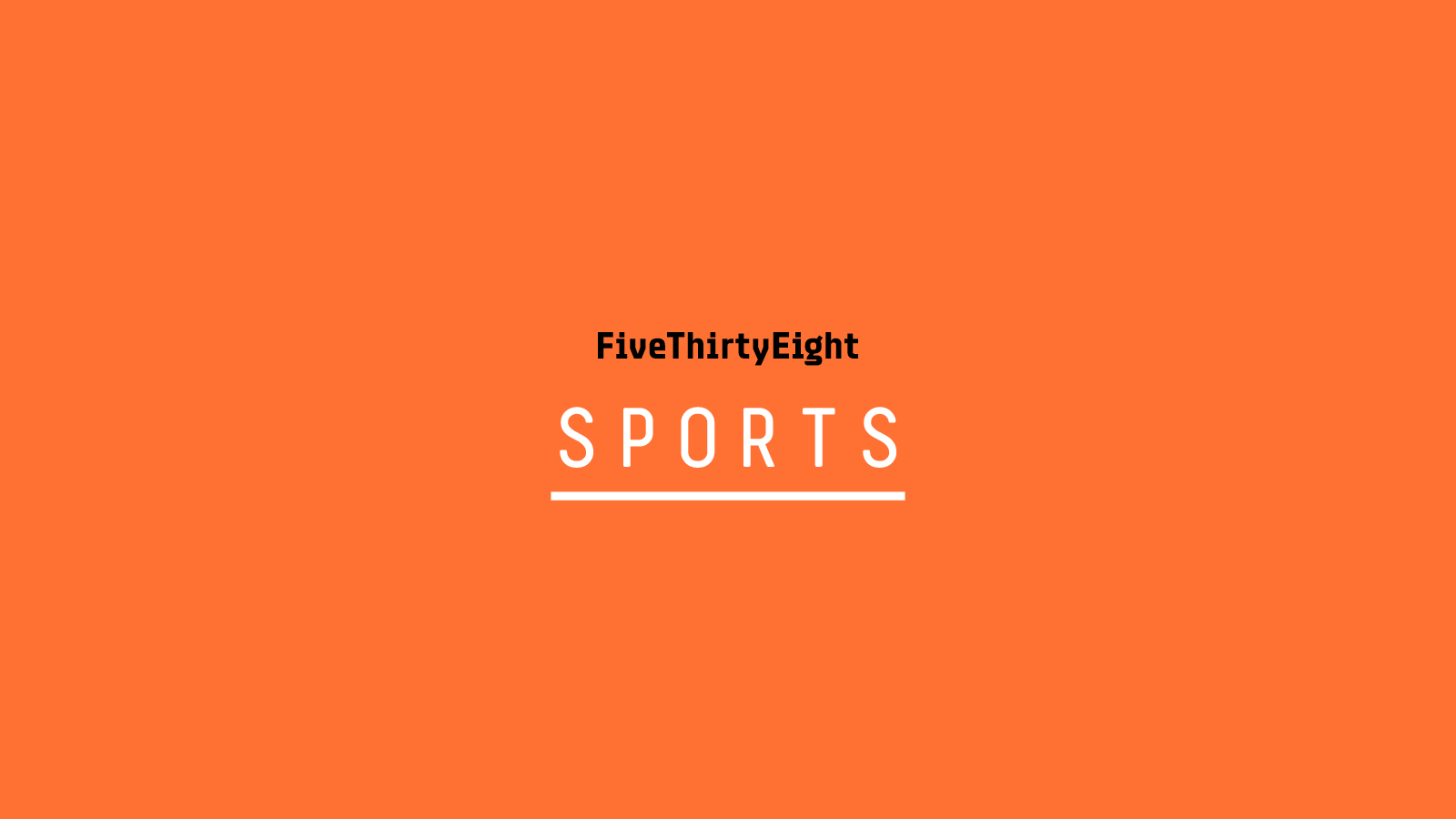
Last Edit
Filed under
Methodology (124 posts)
Brian Burke’s Excitement Index / DonBest injury report / ESPN’s BPI / Sports-Reference.com
Jeff Sagarin’s ratings / Joel Sokol’s LRMC ratings / Ken Massey’s ratings / Ken Pomeroy’s ratings / Sonny Moore’s ratings (men, women)
We’ve been issuing probabilistic March Madness forecasts in some form since 2011, when FiveThirtyEight was just a couple of people writing for The New York Times. Initially, we focused on the men’s NCAA Tournament, publishing a table that gave each team’s probability of advancing deep (or not-so-deep) into the tournament. Over the years, we expanded to forecasting the women’s tournament as well. And since 2016, our forecasts have updated live, as games are played. Below are the details on each step that we take — including calculating power ratings for teams, win probabilities for each game and the chance that each remaining team will make it to any given stage of the bracket.
Our men’s model is principally based on a composite of six computer power ratings:
Each of these ratings has a strong track record in picking tournament games. We shouldn’t make too much of the differences among them: They are all based on the same basic information — wins and losses, strength of schedule, margin of victory — computed in slightly different ways. We use six systems instead of one, however, because each system has different features and bugs, and blending them helps to smooth out any rough edges. (Those rough edges matter because even small differences can compound over the course of a single-elimination tournament that requires six or seven games to win.)
To produce a pre-tournament rating for each team, we combine those computer ratings with a couple of human rankings:
These rankings have some predictive power — if used in moderation. They make up one-fourth of the rating for each team; the computer systems are three-fourths.
It’s not a typo, by the way, to say that we look at preseason rankings. The reason is that a 30- to 35-game regular season isn’t all that large a sample. Preseason rankings provide some estimate of each team’s underlying player and coaching talent. It’s a subjective estimate, but it nevertheless adds some value, based on our research. If a team wasn’t ranked in either the AP or coaches’ polls, we estimate its strength using the previous season’s final Sagarin rating, reverted to the mean.
To arrive at our FiveThirtyEight power ratings, which are a measure of teams’ current strength on a neutral court and are displayed on our March Madness predictions interactive graphic, we make two adjustments to our pre-tournament ratings.
The first is for injuries and player suspensions. We review injury reports and deduct points from teams that have key players out of the lineup. This process might sound arbitrary, but it isn’t: The adjustment is based on Sports-Reference.com’s win shares, which estimate the contribution of each player to his team’s record while also adjusting for a team’s strength of schedule. So our program won’t assume a player was a monster just because he was scoring 20 points a game against the likes of Abilene Christian and Austin Peay. The injury adjustment also works in reverse: We review every team to see which are healthier going into the tournament than they were during the regular season.
The second adjustment takes place only once the tournament is underway. The FiveThirtyEight model gives a bonus to teams’ ratings as they win games, based on the score of each game and the quality of the opponent. A No. 12 seed that waltzes through its play-in game and then crushes a No. 5 seed may be much more dangerous than it initially appeared; our model accounts for this. On the flip side, a highly rated team that wins but looks wobbly against a lower seed often struggles in the next round, we’ve found.
When we forecast individual games, our model normally applies a third and final adjustment to our ratings, for travel distance. Are you not at your best when you fly in from LAX to take an 8 a.m. meeting in Boston? The same is true of college basketball players. In extreme cases (a team playing very near its campus or traveling across the country to play a game), the effect of travel can be tantamount to playing a home or road game, despite being on an ostensibly neutral court. This final adjustment gives us a team’s travel-adjusted power rating, which is then used to calculate its chance of winning that game. However, for the 2021 tournament, we have removed the travel adjustments Model tweak
March 14, 2021 from our model.
We calculate power ratings for the women’s tournament in much the same way as we do for the men’s. However, because of the relative lack of data for women’s college basketball — a persistent problem when it comes to women’s sports — the process has a few differences:
Once we have power ratings for every team, we need to turn them into a forecast — that is, the chance of every team reaching any round of the tournament.
Most of our sports forecasts rely on Monte Carlo simulations, but March Madness is different; because the structure of the tournament is a single-elimination bracket, we’re able to directly calculate the chance of teams advancing to a given round.
We calculate the chance of any team beating another with the following Elo-derived formula, which is based on the difference between the two teams’ travel-adjusted power ratings:
Because a team needs to win only a single game to advance, this formula gives us the chance of a team reaching the next round in the bracket. The probability of a team reaching a future round in the bracket is based on a system of conditional probabilities. In other words, the chance of a team reaching a given round is the chance it reaches the previous round, multiplied by its chance of beating any possible opponent in the previous round, weighted by its likelihood of meeting each of those opponents.
While games are being played, our interactive graphic displays a box for each one that shows updating win probabilities for both teams, as well as the score and the time remaining. These probabilities are derived using logistic regression analysis, which lets us plug the current state of a game into a model to produce the probability that either team will win the game. Specifically, we used play-by-play data from the past five seasons of Division I NCAA basketball to fit a model that incorporates:
The model doesn’t account for everything, however. If a key player has fouled out of a game, for example, the model doesn’t know, and his or her team’s win probability is probably a bit lower than what we have listed. There are also a few places where the model experiences momentary uncertainty: In the handful of seconds between the moment when a player is fouled and the free throws that follow, for example, we use the team’s average free-throw percentage to adjust its win probability. Still, these probabilities ought to do a reasonably good job of showing which games are competitive and which are essentially over.
Also displayed in the box for each game is our “excitement index” (check out the lower-right corner) — that number also updates throughout a game and can give you a sense of when it’ll be most fun to tune in. Loosely based on Brian Burke’s NFL work, the index is a measure of how much each team’s chances of winning have changed over the course of the game.
The calculation behind this feature is the average change in win probability per basket scored, weighted by the amount of time remaining in the game. This means that a basket made late in the game has more influence on a game’s excitement index than a basket made near the start of the game. We give additional weight to changes in win probability in overtime. We also add a bonus for games that spend a large proportion of their time with an upset on the horizon, weighted by how big the upset would be. Model tweak
March 17, 2019 Values range from 0 to 10, although they can exceed 10 in extreme cases.
If you’ve been a FiveThirtyEight reader for really any length of time, you probably know that we’re big fans of Elo ratings. We’ve introduced versions for the NBA and the NFL, among other sports. Using game data from ESPN, Sports-Reference.com and other sources, we’ve also calculated Elo ratings for men’s college basketball teams dating back to the 1950s and for women’s teams since 2001. Our Elo ratings are one of the six computer rating systems used in the pre-tournament rating for each men’s team and one of four systems for each women’s team.
Our methodology for calculating these Elo ratings is very similar to the one we use for the NBA. Elo is a measure of a team’s strength that is based on game-by-game results. The information that Elo relies on to adjust a team’s rating after every game is relatively simple — including the final score and the location of the game. (As we noted earlier, college basketball teams perform significantly worse when they travel a long distance to play a game.)
It also takes into account whether the game was played in the NCAA Tournament. We’ve found that historically, there are actually fewer upsets in the tournament than you’d expect from the difference in teams’ Elo ratings, perhaps because the games are played under better and fairer conditions in the tournament than in the regular season. Our Elo ratings account for this and weight tournament games slightly higher than regular-season ones.
Because Elo is a running assessment of a team’s talent, at the beginning of each season, a team gets to keep its rating from the end of the previous one, except that we also revert it to the mean. The wrinkle here, compared with our NFL Elo ratings, is that we revert college basketball team ratings to the mean of the conference.
While we make no guarantee that you’ll win your pool if you use our system, we think it’s done a pretty good job over the years. Hopefully, you’ll have fun using it to make your picks, and it will add to your enjoyment of both NCAA tournaments.
Editor’s note: This article is adapted from previous articles about how our March Madness predictions work.
Jay Boice A computational journalist for FiveThirtyEight.
Nate Silver Editor in chief.
1.4 Added women’s Elo model and started adjusting excitement index for upsets.
1.3 Added live win probabilities and men’s Elo model.
1.2 Added women’s forecast.
1.1 Started accounting for strength of schedule when making injury adjustments; started using reverted Sagarin ratings as preseason ratings.
1.0 Forecast launched for the 2014 tournament.
Jay Boice was a computational journalist for FiveThirtyEight. @jayboice
Nate Silver founded and was the editor in chief of FiveThirtyEight. @natesilver538
Filed under
Methodology (124 posts)
© 2024 ABC News Internet Ventures. All rights reserved.