Sports
Guide to Building a College Basketball Machine Learning Model in Python – Towards Data Science
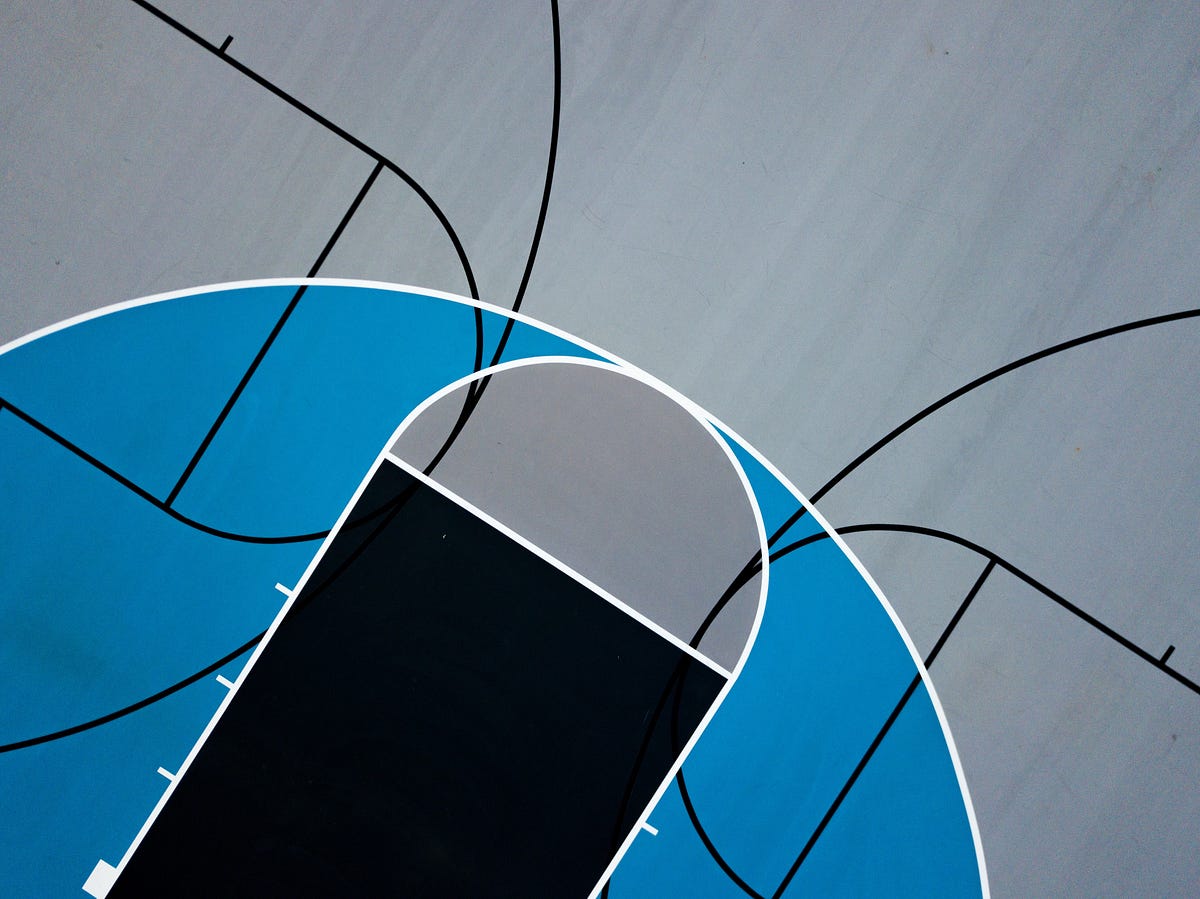
Sign up
Sign in
Sign up
Sign in
Member-only story
Blake Atkinson
Follow
Towards Data Science
—
2
Share
Over the Thanksgiving holiday, I had some free time and stumbled upon a great Python public API created by Robert Clark. The API allows users to pull about any statistic for major American sports very easily from sports-reference.com. Often the hardest part of any data science work is gathering and cleaning data. While some work still has to be done, I’m naturally attracted to any project where the hardest parts have been made easy. My goal is to quickly create a model that will approach the quality of well-known publicly available systems. Gambling markets are really hard to beat, and they will provide a good measuring stick for accuracy. Although early season college basketball is often cited as a soft market, it’s very unlikely this model would be profitable in Vegas — there are a few key elements outside the scope of it. My code for this project can be found on Github.
My plan is to find the most important statistics relevant to predicting college basketball outcomes. Then I can leverage two powerful models, one Light Gradient Boosting Machine (LGBM), and one neural network to predict college basketball spreads…
—
—
2
Towards Data Science
M.S. in Data Science, mostly sports things, @blaketatkinson on twitter, open for new work
Help
Status
About
Careers
Press
Blog
Privacy
Terms
Text to speech
Teams